AI – no other promise has sparked human imagination more than the prospect of creating a new artificial form of intelligence. And rightfully so, Large Language and other AI models have and will have a dramatic impact on a lot of important areas like science, education, and of course eCommerce. So it’s no wonder that eCommerce technology vendors are using the label AI with the same frequency as Sundar Pichai at the last Google I/O.
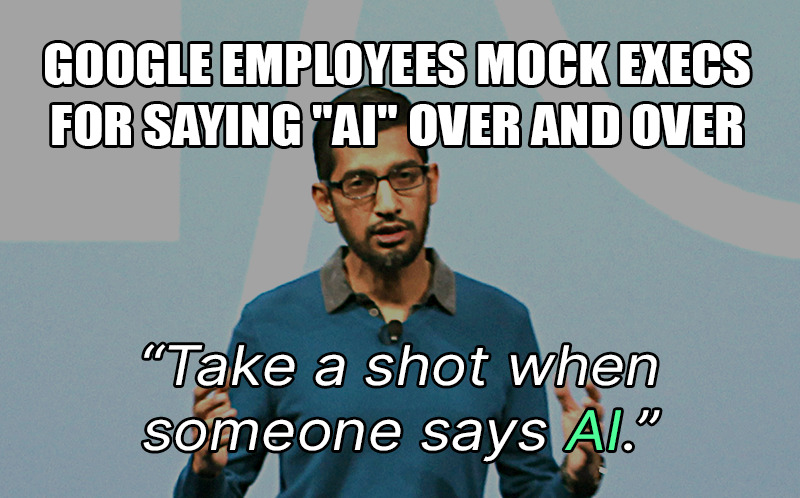
The problem is that it‘s much easier to talk about AI than actually creating a powerful model that integrates into your solution in an impactful way. That’s why we developed this blog post with a no bullish!t methodology – 100% factual and in collaboration with the smartest brains at FactFinder (our AI researchers and developers), to give you genuine insights into how our AI, Atlas, is different and why it can make a big impact on your eCommerce goals.
But before we get into that, let’s explore why other models have struggled to live up to their promises.
Why product discovery is a hard problem for AI
Thinking from first principles, breaking down eCommerce to its most basic level, it’s nothing more than matching demand and supply in a shop on the internet. Easy right? In a perfect world, consumers should specify what they want, and the shop would show the best possible fit for their needs. Unfortunately, it’s not that simple. There are hundreds of problems to retrieve and rank the best possible products, which, according to The Harris Poll, cost online shops $300 billion a year alone in the US.
Current AI models can solve these problems only to some degree due to 3 main reasons:
- Search queries are difficult to understand for a machine
Modeling language is not an easy task in eCommerce. There are many reasons for this including typos, homonyms (e.g. fan has two meanings), words with the same basis (e.g. water and watermelon), shoppers using different words to describe the same item (e.g. mobile phone and smartphone) and so on.
- Sparse interactional data is not suitable for real 1:1 personalization
Most customers only make a handful of purchases in a particular shop. In the context of thousands or millions of products, it’s extremely difficult for an AI model to draw any conclusions from this to personalize the experience to the individual, due to a lack of interaction data. For example, if you bought a toaster in April and a pillow in October this doesn’t provide the AI with enough data to accurately predict your next purchase.
- Personalization by segmentation doesn’t work
This lack of data (Problem 2) is why most personalization solutions are trying to create shopper segments that behave the same. The problem is that we live in an individualized world and demographic data is not the best predictor of preferences. As you can see in the image below King Charles and Ozzy Osbourne would fall in the same segment – and it’s unlikely they buy the same stuff.
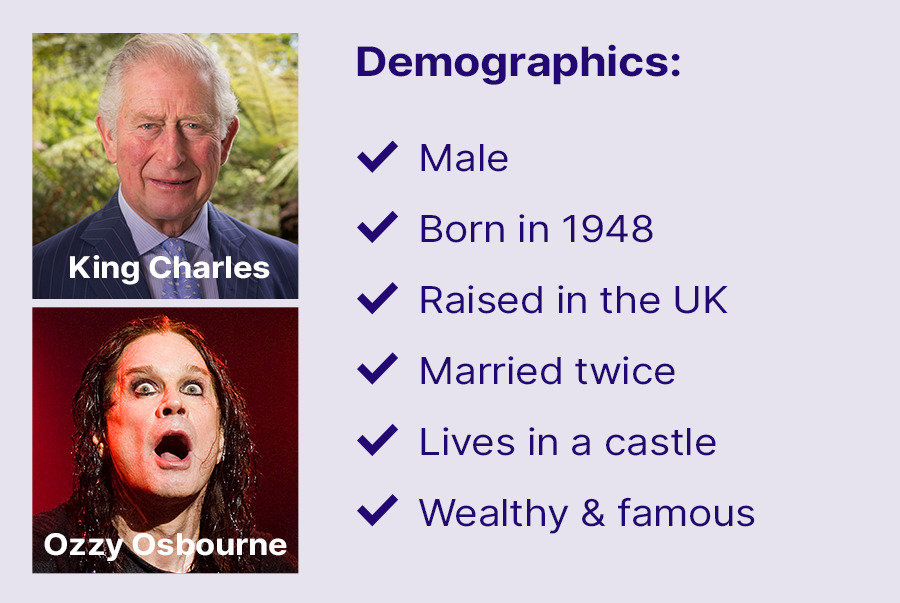
Truly understanding your customers is hard. The upside is that companies that have the AI technology that can overcome these challenges and understand their shoppers needs, see significant improvements in their onsite conversion for every visitor entering their shop – yielding growth and higher profitability. So these are problems worth solving.
“We ourselves are surprised at how quickly the switch to FactFinder has been reflected in sales figures, as our search-generated conversion rate has increased by 20 percent since go-live.” – David Büschler, Online Marketing Manager Globus Baumarkt
How Atlas AI is unique – an inverted approach to product discovery
We have approached AI in a different way – we inverted the problem. While all other solutions focus on modeling language (understanding the query and matching it with the assortment). Our AI focuses on learning your products so that it gains a human-like understanding of your assortment. This means that the queries and interactions from shoppers can be processed in a much smarter way.
“It really works to tackle much of life by inversion where you just twist the thing around backwards and answer it that way. All kinds of problems that look so difficult, if you turn them around, they are quickly solved.” – Charlie Munger, Investor and Philanthropist
We’ve called this unique model Atlas AI, as it creates contextual neural maps of your assortment.
The model is trained with your vectorized product data. This allows us to extract all the features and attributes from a product using a series of embeddings. This training data is used to create a large number of neural networks that act as a detailed representation of your assortment.
Atlas groups products in a similar context together and thereby creates contextual maps of your assortment. Distances between them on the map represent differences between the products in that specific dimension.
The neural maps it creates have several layers so that it is able to understand your products and their relationships in a very granular way. The first layer could be as simple as grouping the products within the same category (eg. Smartphones).
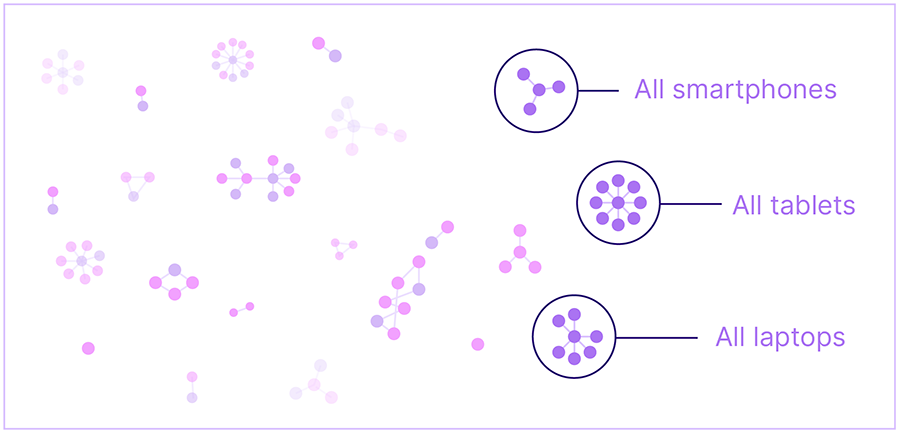
Once the first layer is created the model creates deeper layers (eg. screensize), followed by another (eg. price) and so on until it has a contextual, human-like understanding of your assortment – how deep the model gets depends on your specific catalog.
Now when shoppers enter your shop and search or click on stuff, they are not interacting with your actual products, they are interacting with your Atlas AI model. And as Atlas understands your assortment in a contextual, human-like fashion, it learns from just a single click, solving the sparse interaction data problem mentioned earlier. This is the basis for 1:1 relevance in an unprecedented way.
What are the benefits for online shops?
Based on its contextual understanding of your assortment Atlas AI has 4 main benefits over other solutions:
1. It creates general relevance – automatic, fast and flexible
With your assortment now deeply understood and mapped into detailed neural networks understanding human language and behavior gets a lot easier. So once Atlas has learned how shoppers behave on the site it automatically creates general relevance in your search results. The benefits:
- Reduces the need to manually optimize search results as it learns from behavior which products are relevant for a specific search query. See Gif below – the initial search for iPad brings up mixed results, but as soon as an iPad is viewed and the search is repeated, it learns from just one click what the visitor is looking for. This will influence the search results for all future shoppers as well.
- Adaptability: relevance is flexible so it learns within a few interactions that a helmet search can mean something different in summer (bike helmet) than in winter (climbing helmet)
- eCommerce KPI uplift: The increase in general relevance lets shoppers easily find what they want
2. It understands shoppers’ intent in real-time
Let’s assume someone is searching for an “LG TV” and afterwards searches for the brand “Samsung”. In this case the engine understands the shopper is in the TV section (similar to shopping in a local electronics shop). So in this situation it will respond to the query with TVs vs. showing a general overview of Samsung products. This creates frictionless individual shopping experiences and thereby increases conversion and customer retention.
3. It understands personal preferences in perfect granularity
now assume Lisa buys your most expensive metallic espresso machine from Sage by Heston. This teaches our model a lot of things like Lisa is very price sensitive, she seems to prefer metallic kitchenware and has a preference for the brand Sage by Heston. All this information is understood in the right context which allows for intelligent 1:1 personalization. For example, if Lisa then searches for a toaster whi (kitchen appliances) of the espresso machine the model will apply all that it learned (see Gif below). But if you are coming back and search for bathroom furniture it will keep elements that make sense eg. not very price sensitive and removes elements that don’t make sense necessary like the metallic look. This creates a much stronger 1:1 customer experience and thereby increases conversion, AOVs and customer retention (fully GDPR compliant).
4. It works in harmony with human intelligence
company is that we create AI that works in harmony with eCommerce teams. That’s why we have an easy-to-use UI that allows them to control the AI based on their business goals. At the same time the AI enables eCommerce teams to automate most of their product discovery related tasks, so that they only need to add their human touch and expertise on top.
“We used to spend about 30 hours a month keeping our search up to date. Now we spend a maximum of four hours a month doing that. It’s a big improvement for us because it frees up time and resources for other areas of the business.”
– Joachim de Boer, Co-founder DeOnlineDrogist.nl
How does the recent GPT hype play into this?
It only took ChatGPT 5 days to reach more than 1 million users, making it the fastest-growing online platform – and not without reason: used correctly, Large Language Models (LLMs) have the potential to create value for a large range of tasks and in our context to supercharge eCommerce experiences.
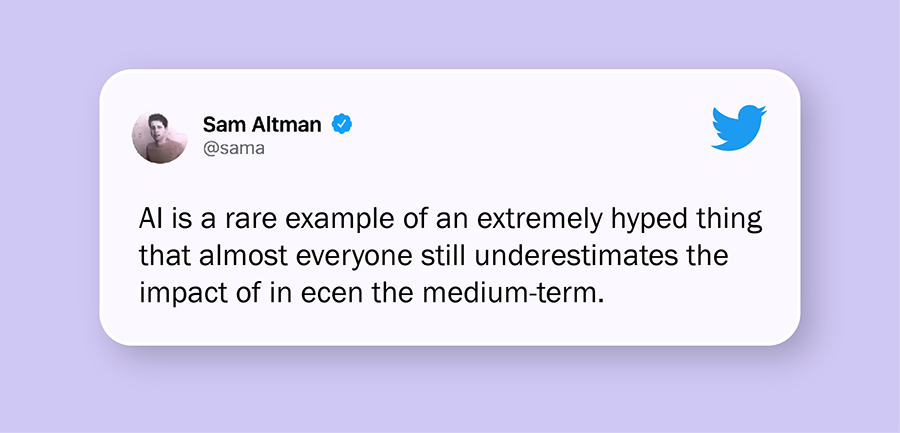
Besides using them to automate tasks in your UI (stay tuned for news on GPT Synonyms) we are currently working on integrating LLMs in the training process of our Atlas AI model. This will make Atlas’s understanding of your assortment super-human-like. Imagine knowing all your products in the deepest granularity, without the need to provide a perfectly comprehensive and structured product data feed. Additionally, we are currently running several tests on how LLMs can be integrated in our retrieval process to:
- give Atlas more context about specific queries further improving the relevance of its output
- enable multimodal search making it possible for shoppers to use images as a query input
There is one last thing… we are super thrilled to announce that the Atlas AI model will be made available in FactFinder Next Generation soon! Stay tuned for more product updates, product discovery is an exciting space at the moment!
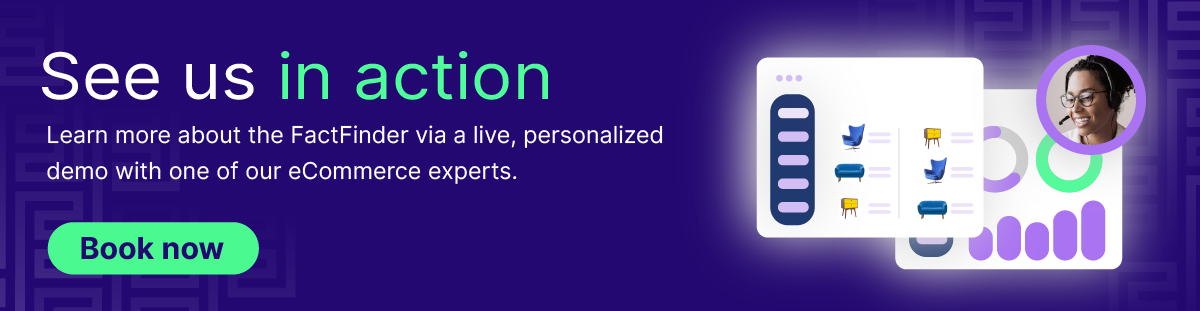